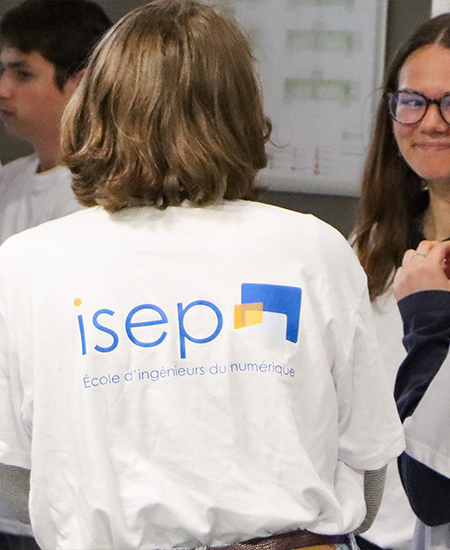
Actus &
Rencontres
L'école
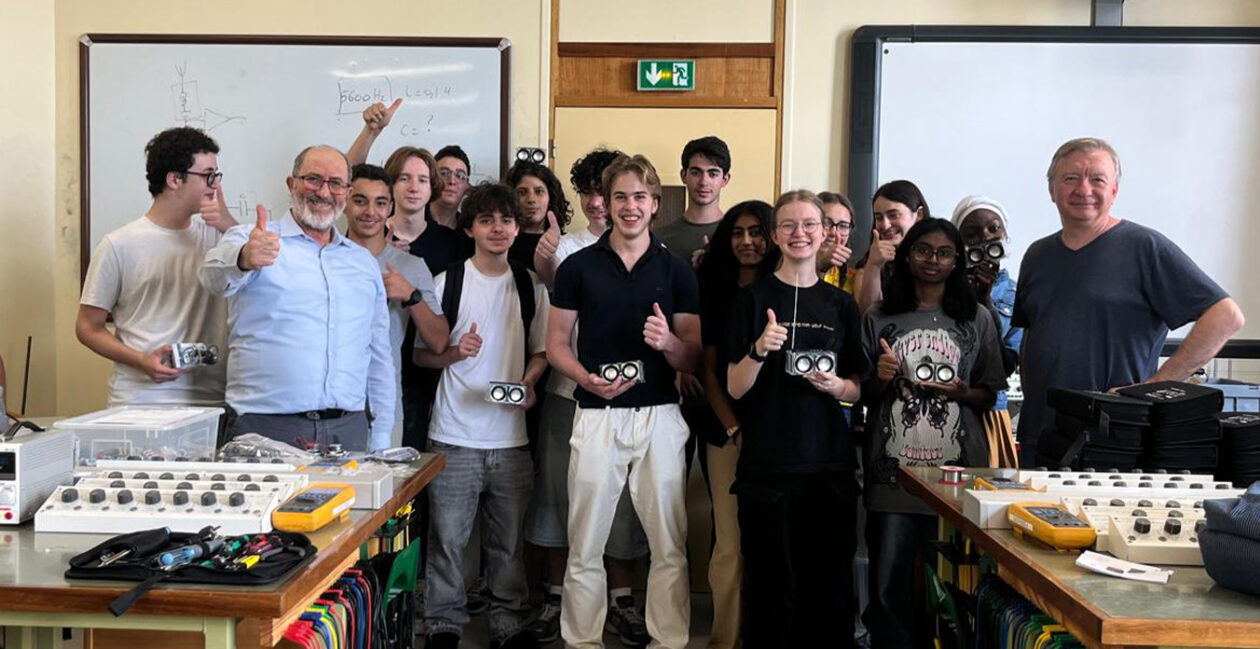
30 juin 2025
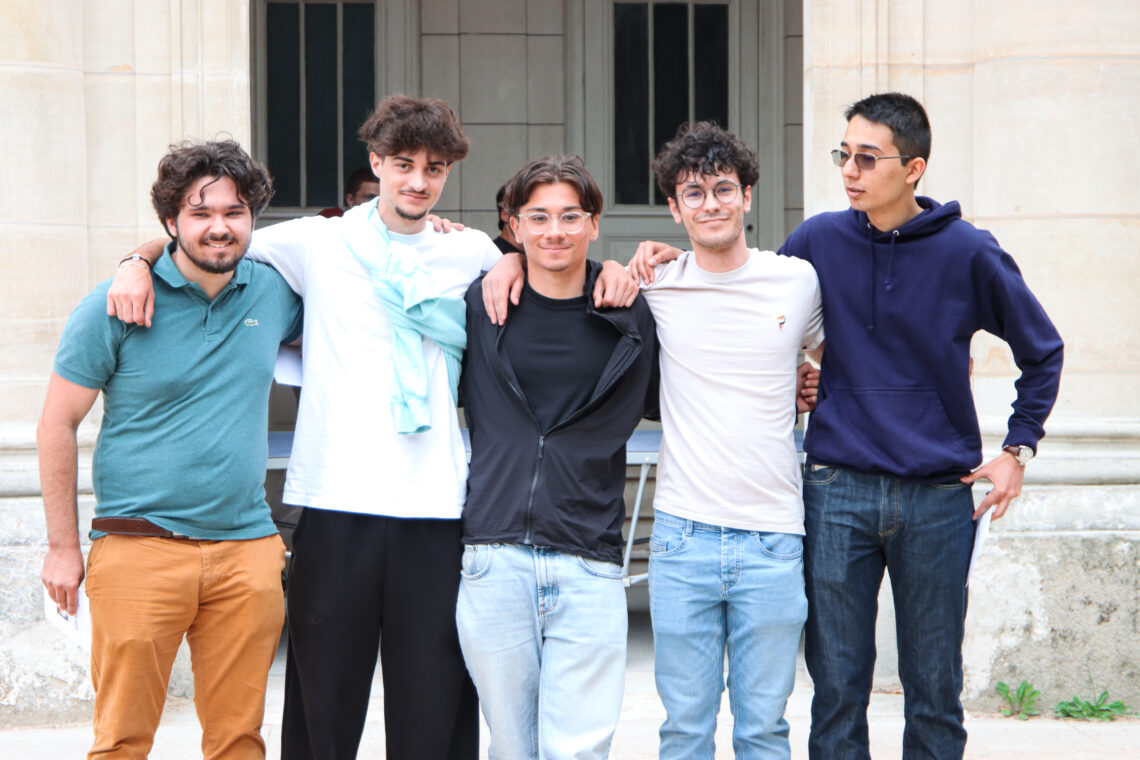
28 mai 2025
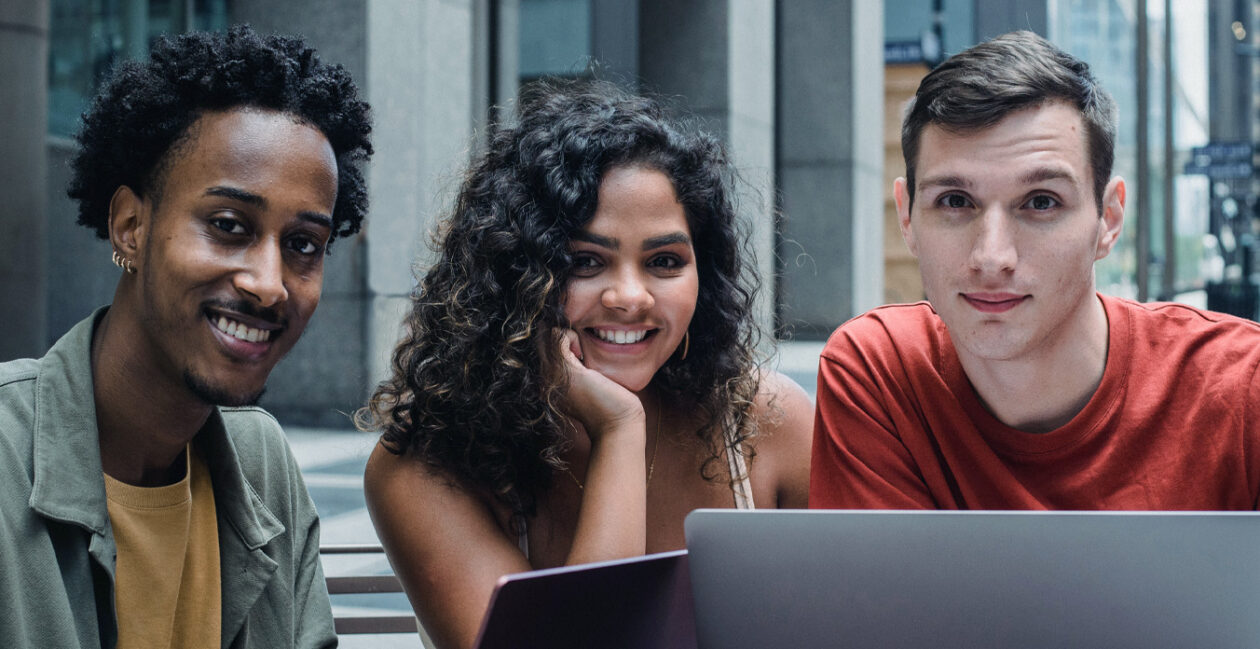
22 mai 2025
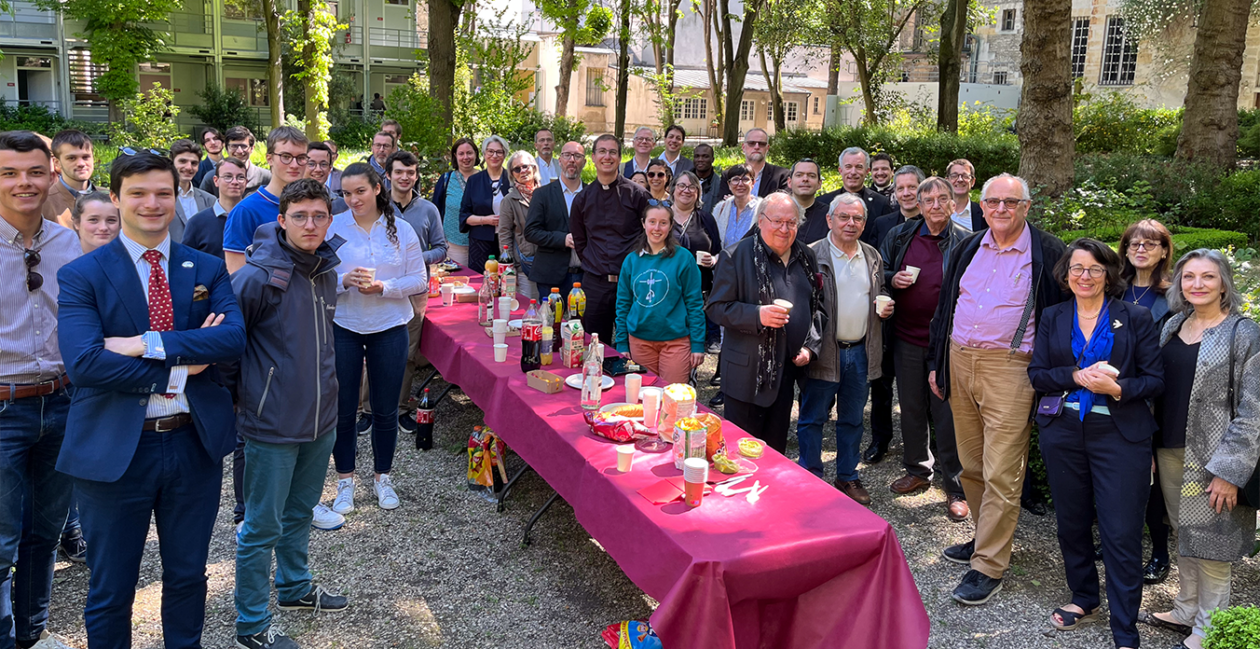
7 mai 2025
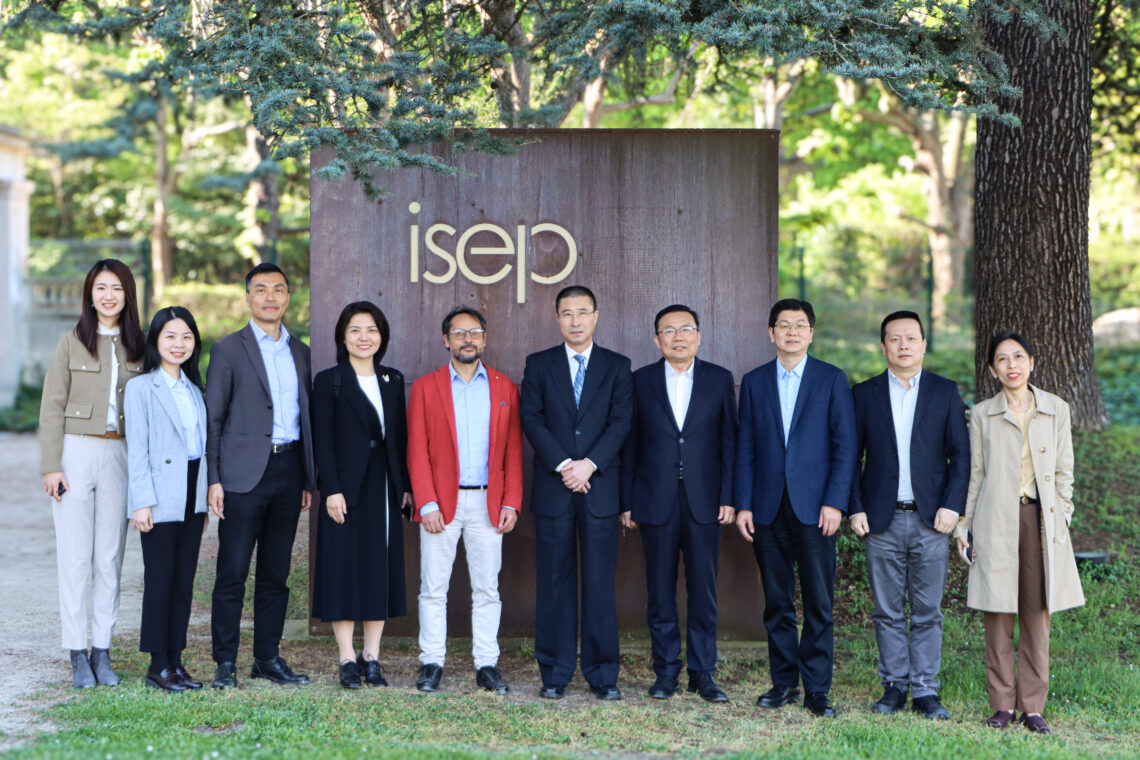
23 avril 2025
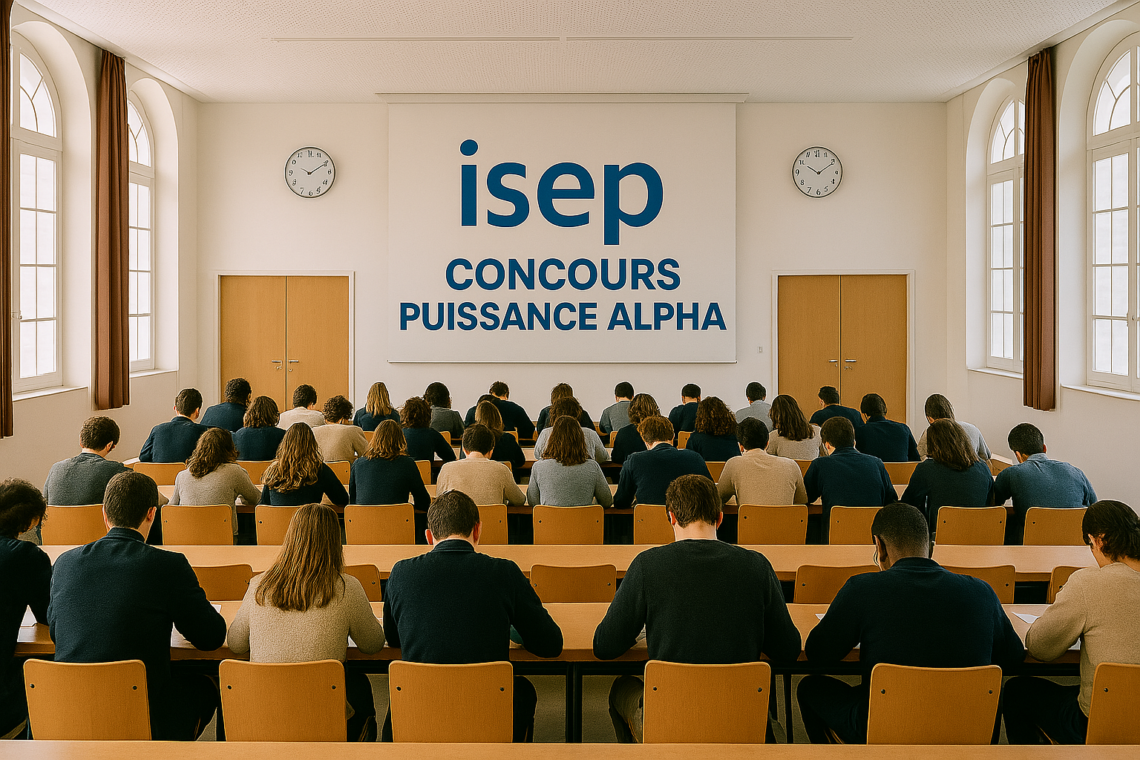
15 avril 2025
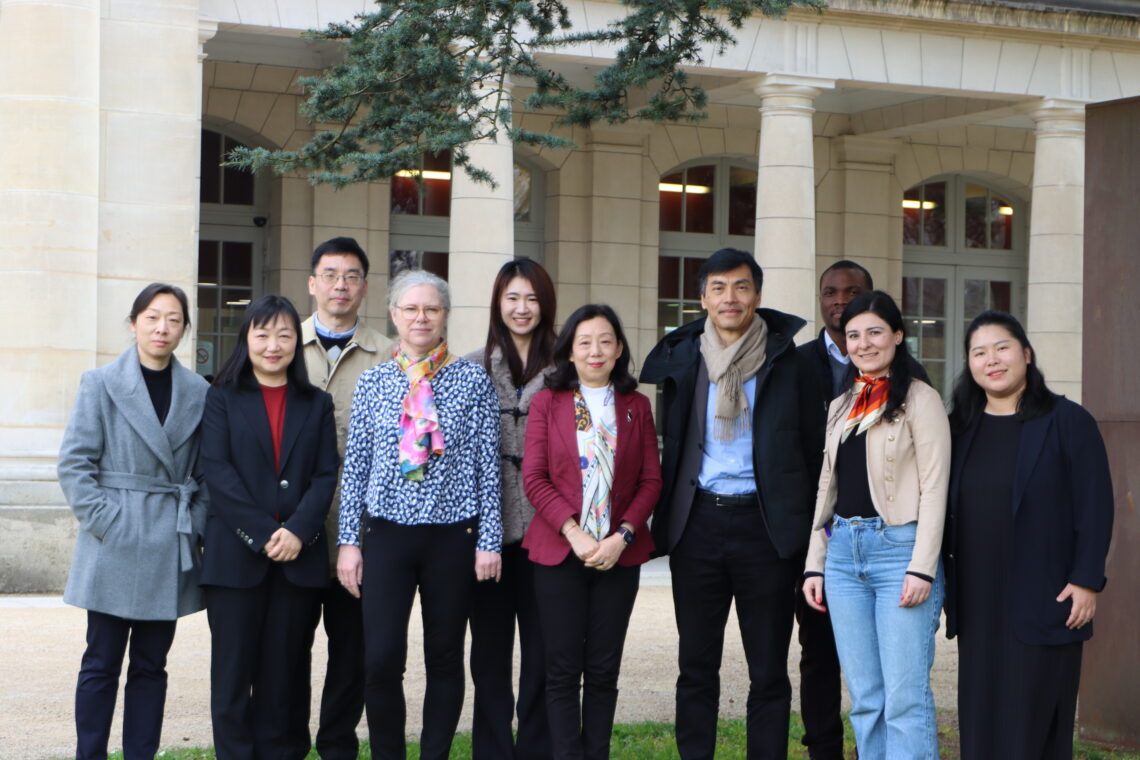
24 mars 2025
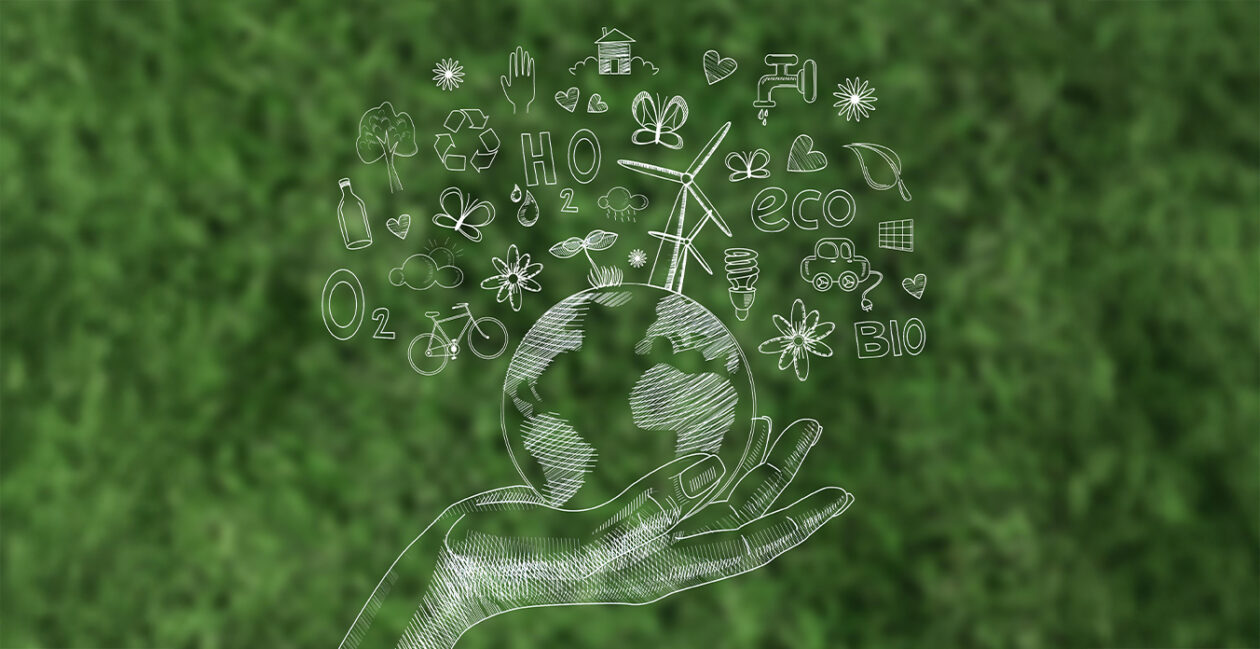
27 février 2025
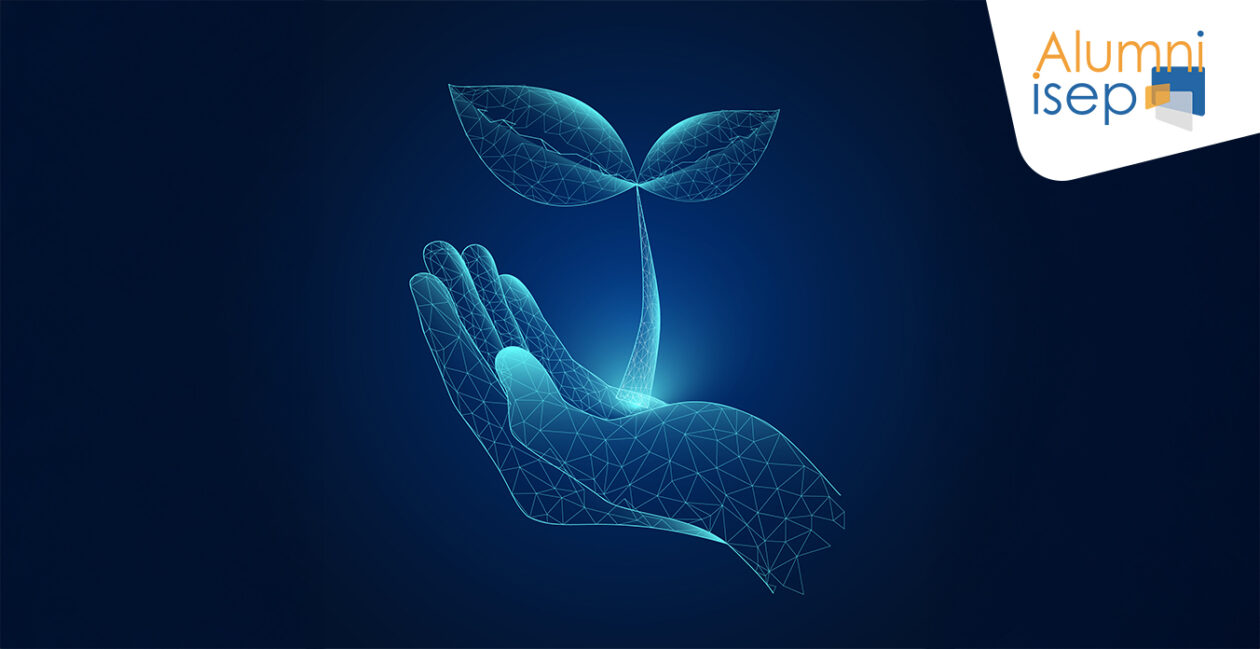
26 février 2025
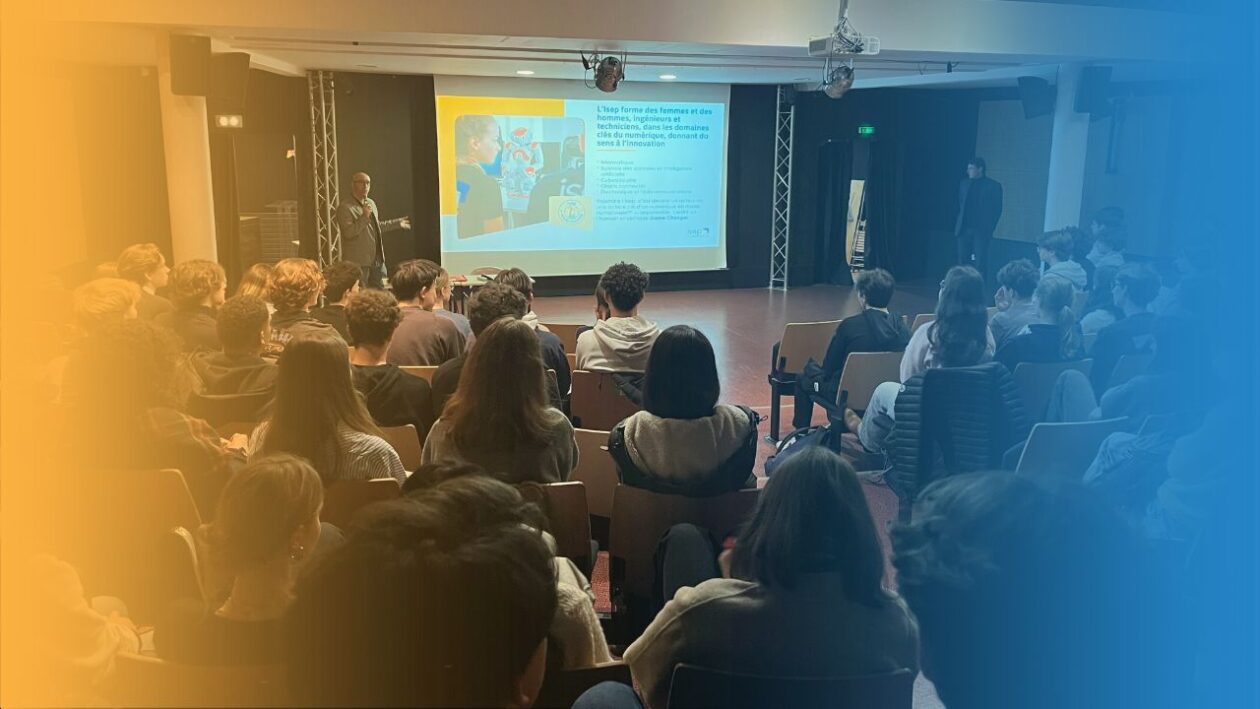
24 février 2025
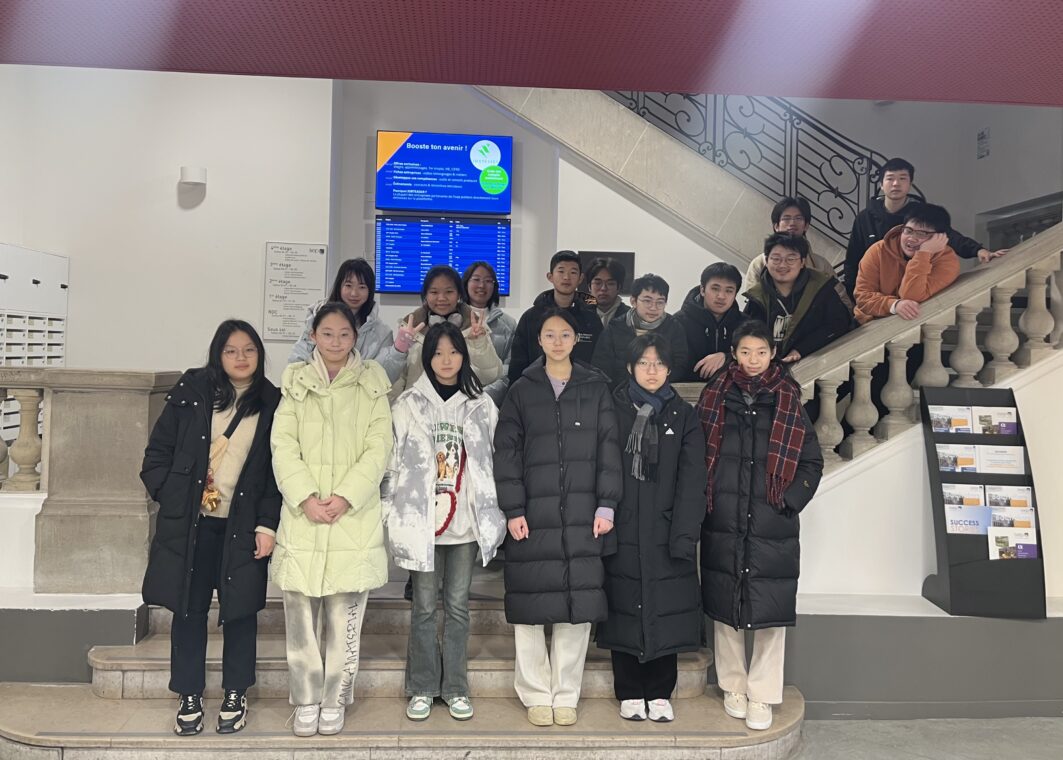
14 février 2025
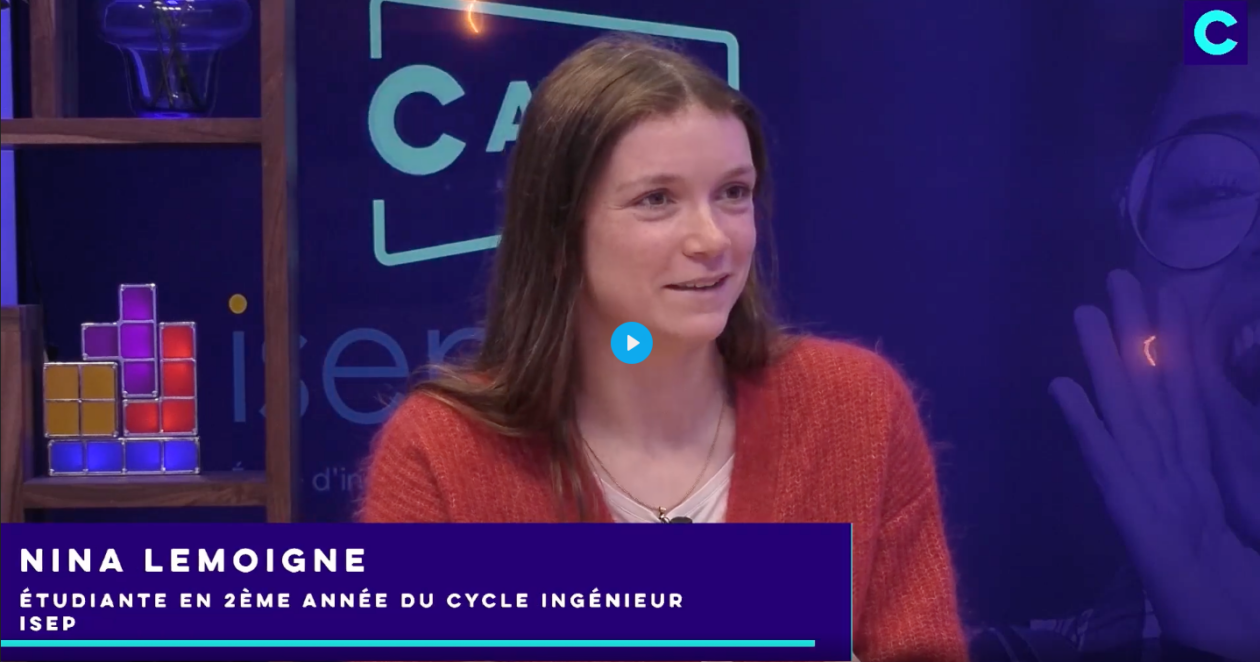
6 février 2025
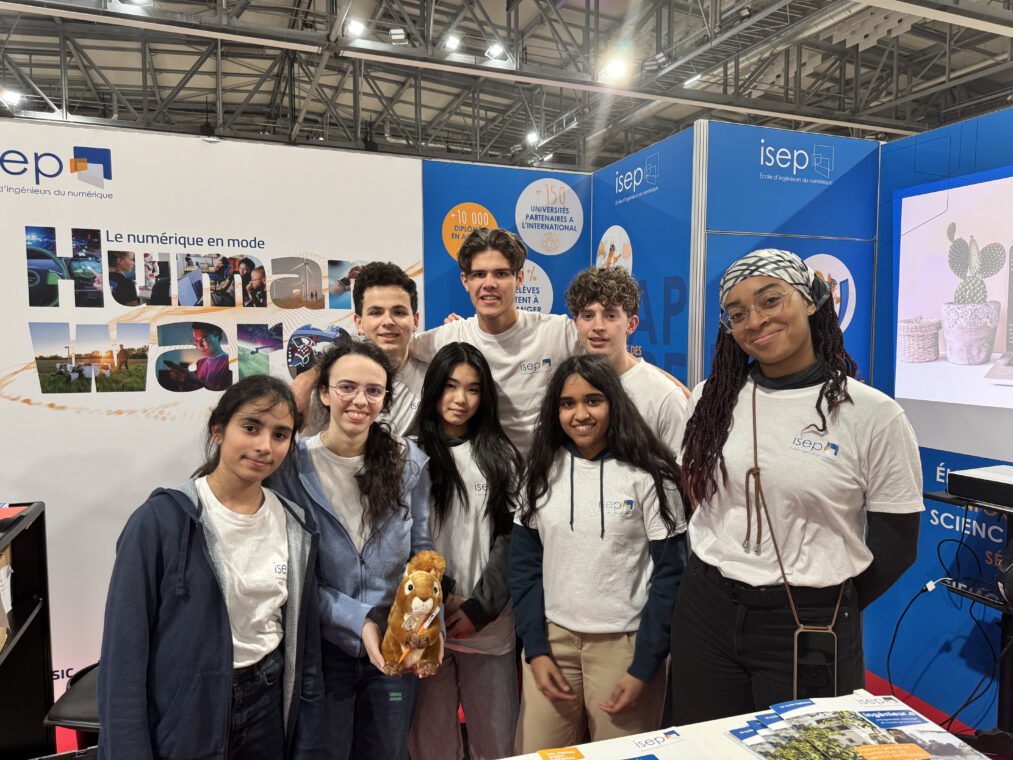
28 janvier 2025
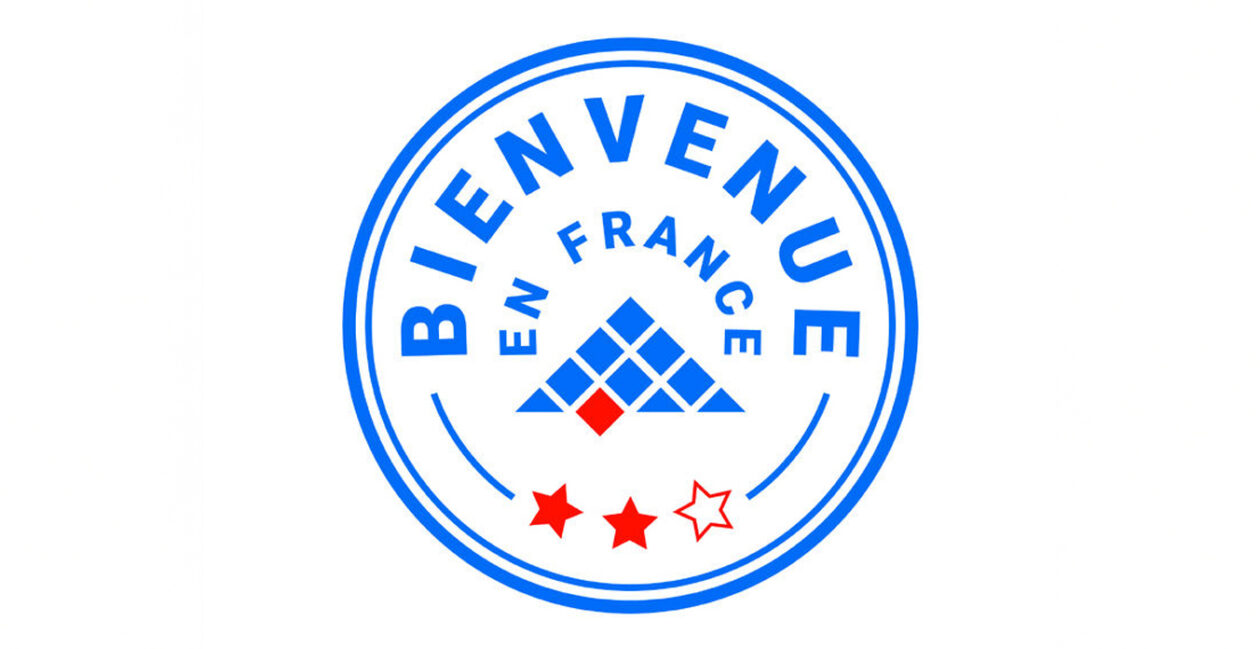
23 janvier 2025
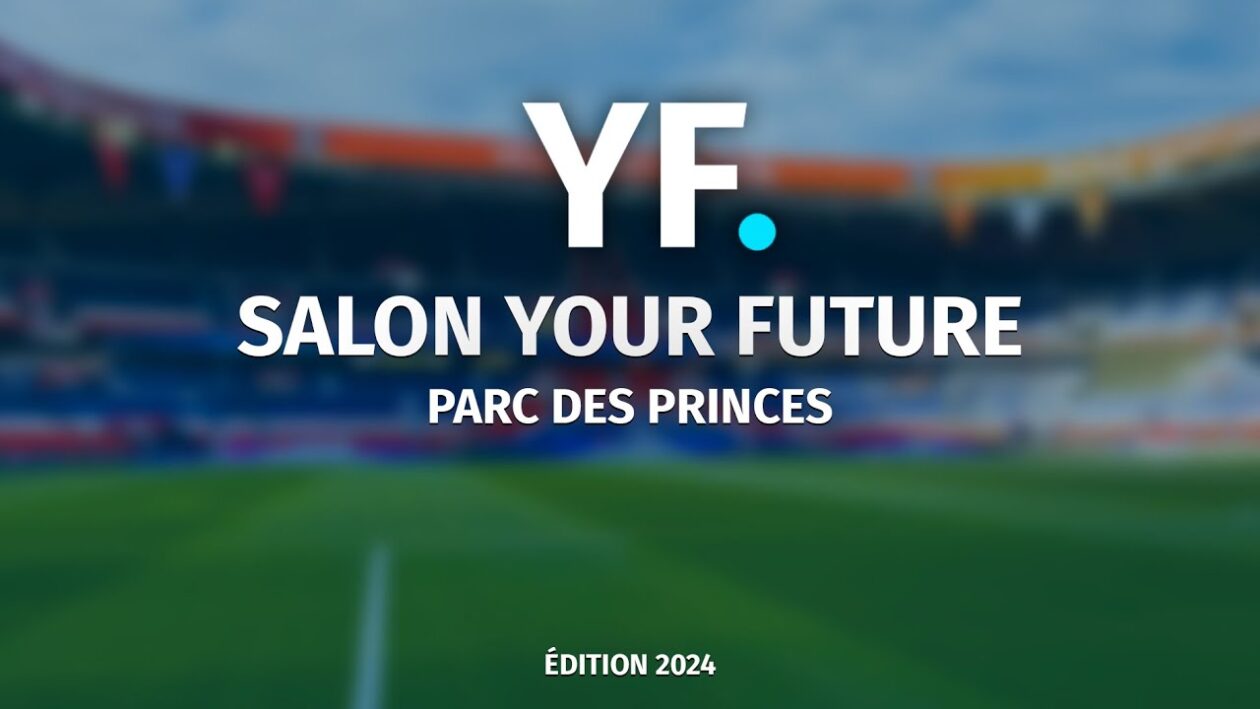
20 janvier 2025
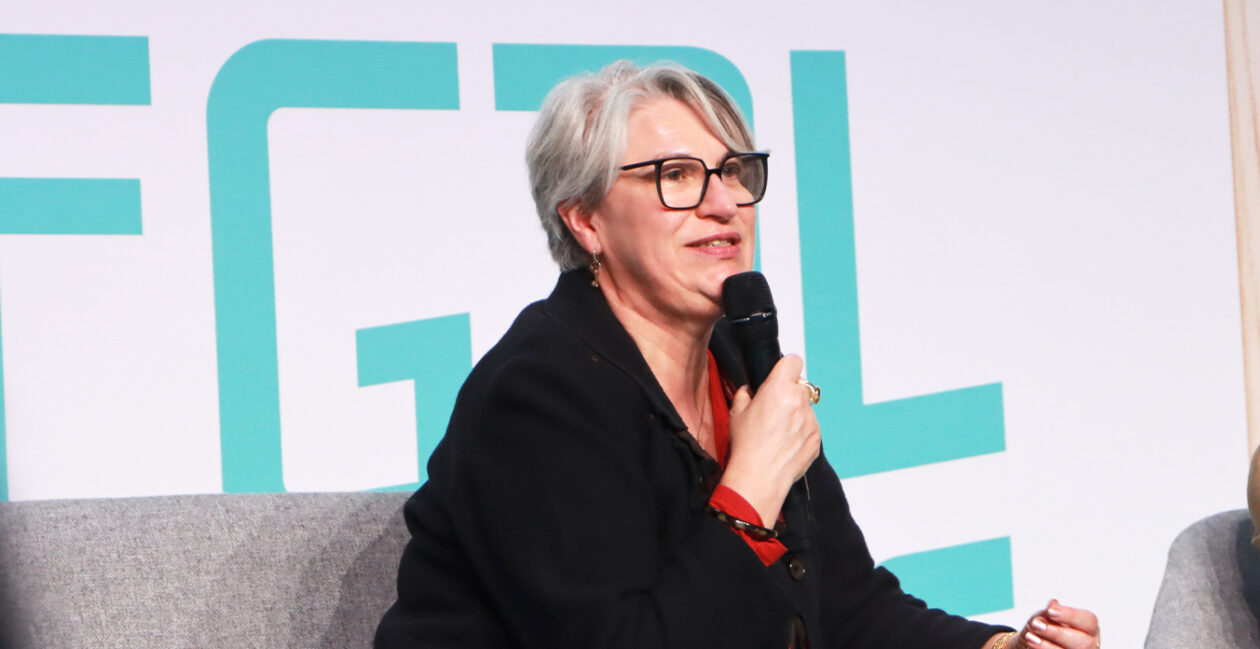
14 janvier 2025
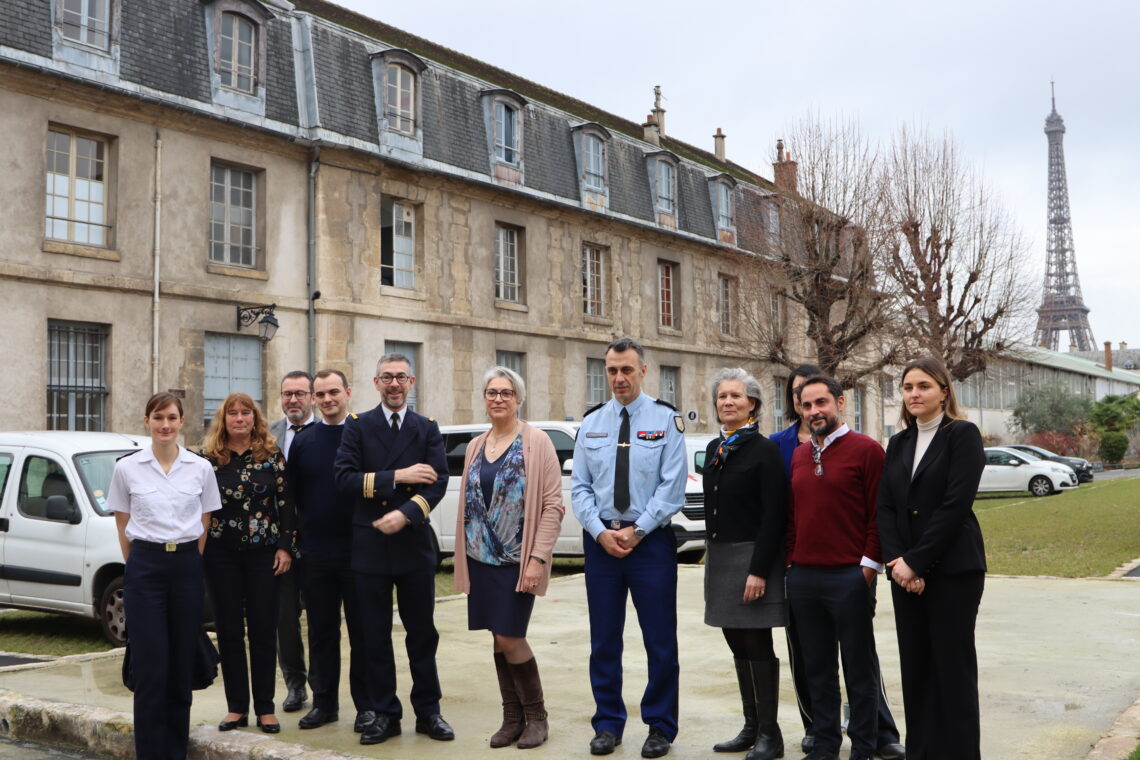
9 janvier 2025
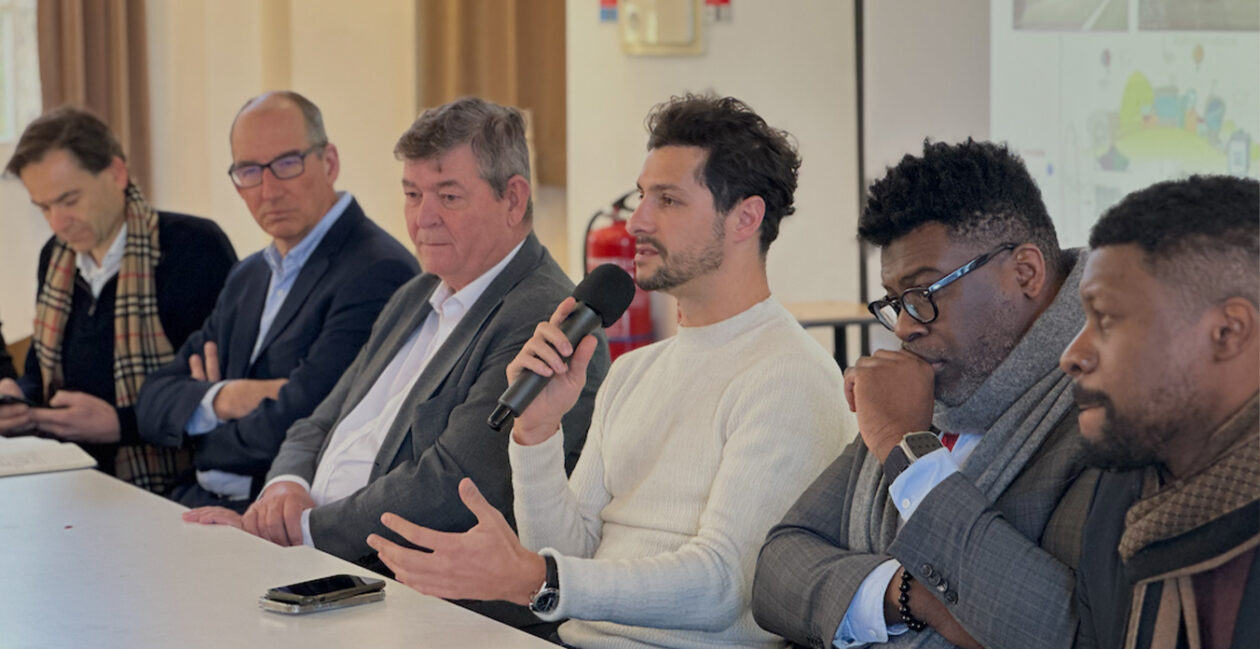
8 janvier 2025
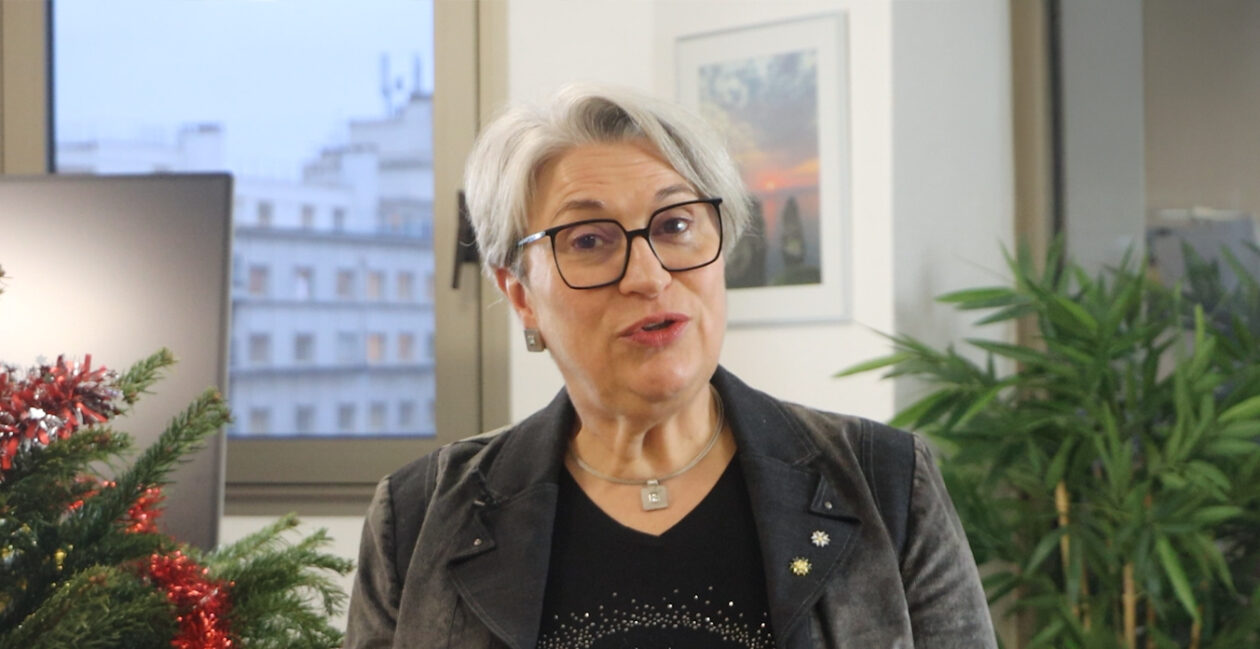
20 décembre 2024
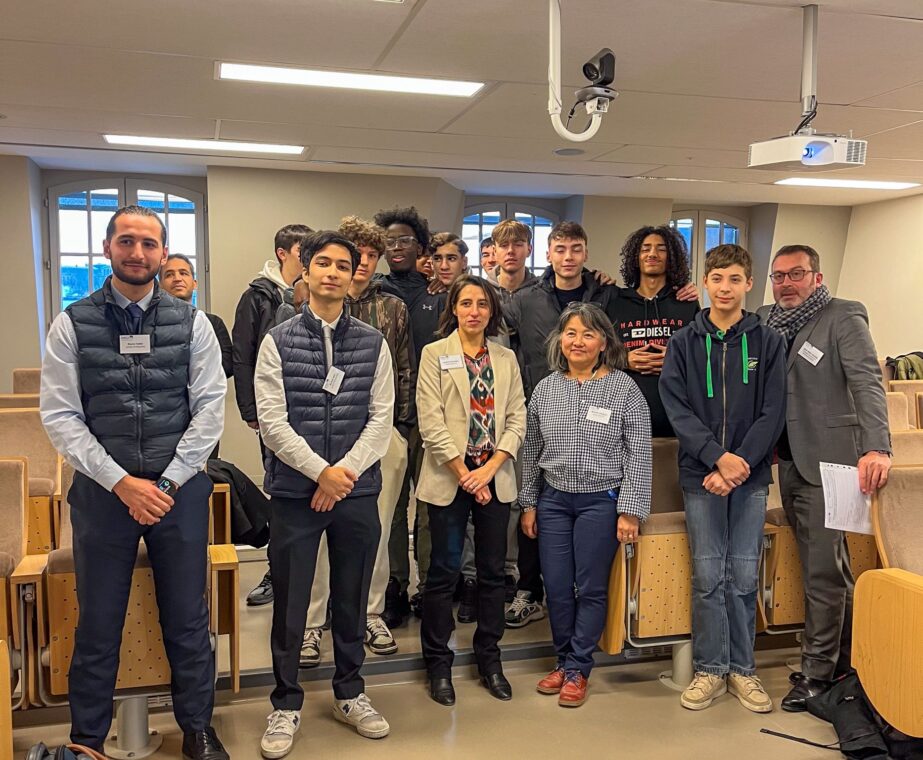
13 décembre 2024
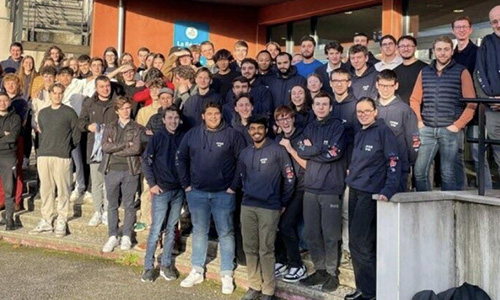
10 décembre 2024
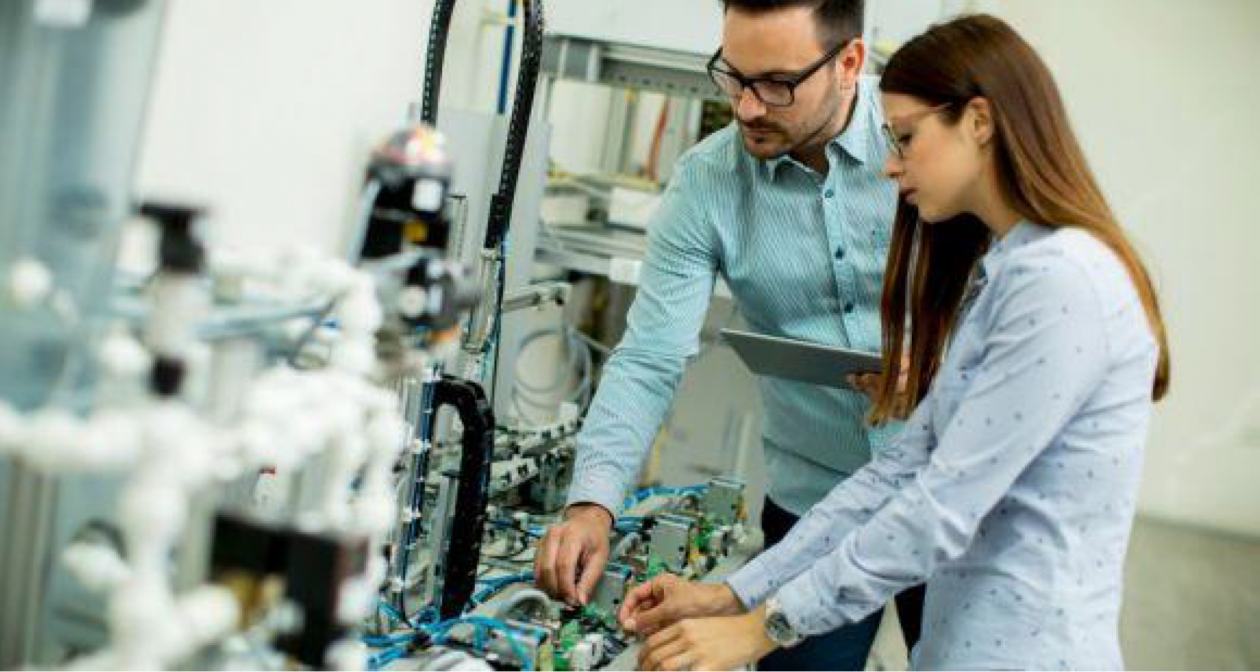
10 décembre 2024
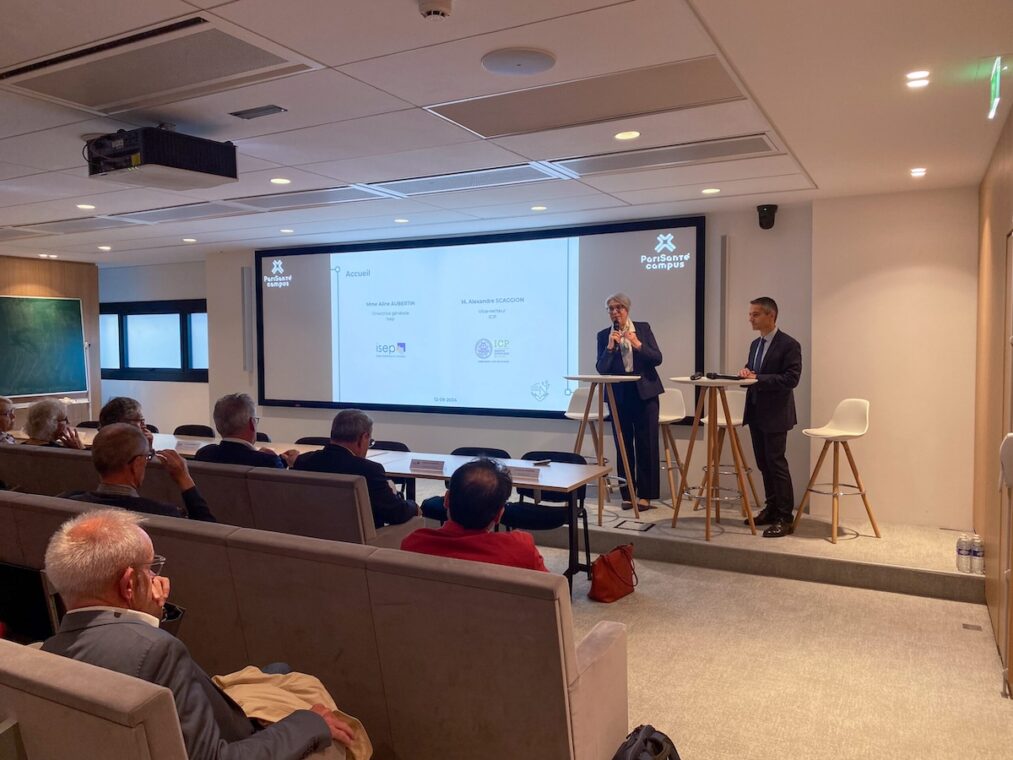
17 septembre 2024
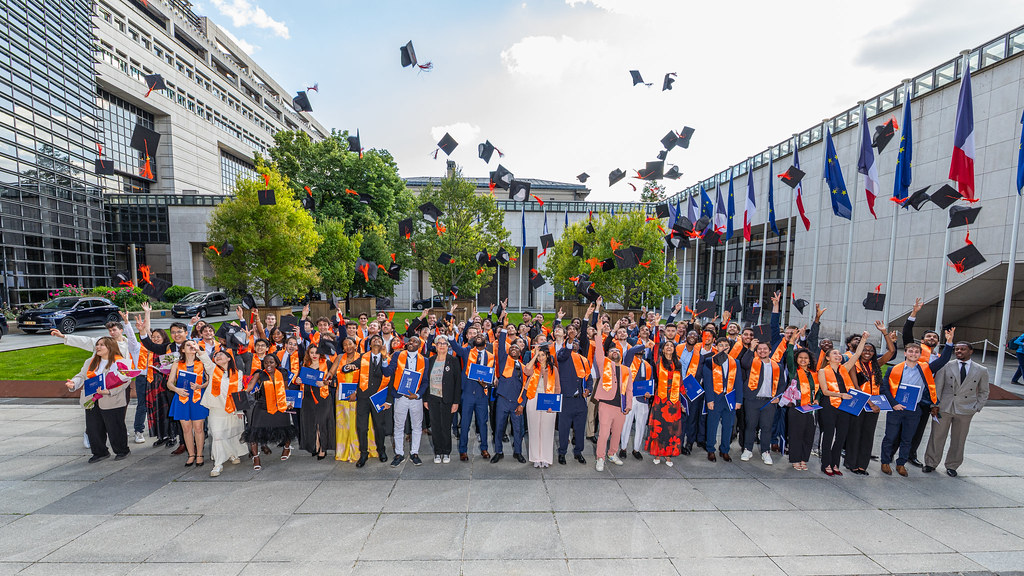
27 mai 2024
Nous rencontrer
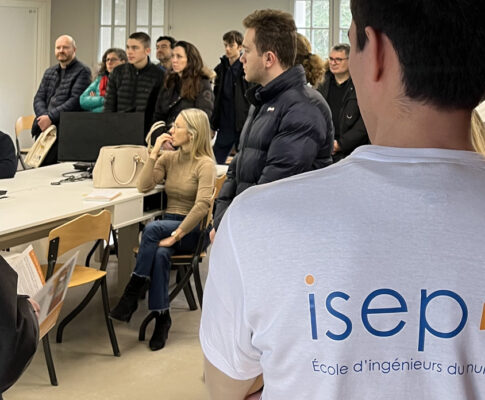
19 juin 2025